1 - Why we wrote this
Artificial intelligence is transforming how organisations operate, and charities risk being left behind. The technology is advancing exponentially while charitable organisations typically move forward through careful, iterative steps. This growing gap between AI capabilities and organisational readiness creates particular challenges for mission-driven organisations, which must balance innovation with responsible stewardship of limited resources.
We wrote this playbook to help charities use AI thoughtfully and effectively. We are drawing from decades of experience working both within charities and alongside them as consultants. Across AI, design, strategy and digital transformation we’ve worked with the likes of RNIB, CRUK, Mencap, Macmillan, YoungMinds, Cruse, Young Lives vs Cancer, Breast Cancer Now, Greenpeace, GOSH charity, the UN and many, many others. But, rare for the sector, we’ve combined this with experience working with, and inside, corporations like Google and Dyson. Our range of experience has given us unique insight into how technology adoption can serve charitable missions.
The rapid pace of AI development shows no signs of slowing. As capabilities expand and costs decrease, the technology becomes increasingly accessible to organisations of all sizes. For charities, this presents an opportunity to enhance their impact and extend their reach, but only if they can implement AI in a way that aligns with their values and operational realities.
We can see this rapid pace of AI with fundraising teams in particular. Commercial organisations are already using AI to personalise outreach and optimise campaigns but many charities are still using traditional approaches that may become increasingly less effective. This gap risks putting charitable fundraising at a disadvantage just at the moment where they could increase their impact and precisely when many causes need support more than ever.
The rapid pace of AI development shows no signs of slowing. As capabilities expand and costs decrease, the technology becomes increasingly accessible to organisations of all sizes.
The aim of this playbook is to distil practical, actionable guidance grounded in real-world experience. We're keenly aware that using AI requires a delicate balance. We are as uneasy as others about some of the bad outcomes that AI can cause. Rather than promoting hype, our aim is to offer a structured path forward that helps charitable organisations navigate this transition thoughtfully and effectively, ensuring technology serves their mission rather than distracting from it.
Part one: background to AI
2 - What is AI?
If you want to, you can skip this section. This section gives a brief overview of why AI is important and where it’s come from. Chances are though, if you’re like most charity leaders we’ve worked with, you already know that AI is important and are just looking for practical solutions and applications for it. If that’s you then feel free to jump ahead to Foundations where we discuss how you can start making sense of AI for your charity. For everyone else let’s go on a quick tour of artificial intelligence’s origins.
It's not new
Artificial Intelligence (AI) has existed as a discipline since the 1950s. The origins arguably go back further to ideas like the Mechanical Turk, but its Dartmouth College in 1956 where it emerged as a discipline. John McCarthy, Marvin Minsky, Nathaniel Rochester, and Claude Shannon were the drivers behind the idea and were nothing if not ambitious. They believed that "every aspect of learning or any other feature of intelligence can in principle be so precisely described that a machine can be made to simulate it." More simply, they believed that anything that typically required human intelligence could be done by machines.
Since then different forms of AI have entered our lives. At the most basic that might be algorithms that choreograph traffic lights through to more recent, and more complex, generative AI tools like ChatGPT. It was the launch of ChatGPT in November 2022 when it really burst into popular consciousness - with the fastest ever growth curve in history - but it’s been since the 2010s that it has started to become clear that the original ideas from the Dartmouth workshop might have been viable. That’s been a combination of Moore’s Law making computers ever more powerful, or scientists like Fei-Fei Li (and her ImageNet project) making it clear that machines could complete narrow tasks to almost human-level abilities.
It’s a tapestry of technologies
AI is increasingly being described as a General Purpose Technology. These are technologies like writing, printing, sail-power, steam, electricity, computing or the internet that create new paradigms by being pervasive, improving over time and creating innovation spillovers so that new services and products are possible. Unlike other general purpose technologies AI hasn’t quite matured to the point where you can point to a single definition. It’s a tapestry of technologies like computer vision, natural language processing, expert systems, generative AI and any number of other terms and acronyms that will hurt your head. It is clearly starting to consolidate around machines that can, end-to-end, replicate human intelligence.
It is starting to coalesce, at least for now, around generative AI. Reading the UK government’s recent AI Opportunities Action Plan, the Stargate project from the US government, or looking at where business capital is being spent it’s clear that the energy supply and computing infrastructure being invested in are those based on neural networks.
There are two flavours
To try and simplify things you can think of there being two flavours of AI.
Deterministic AI
Deterministic AI is predictable AI. In computer science this is generally referred to as Symbolic AI. It is a system that follows a set of explicit rules, or symbols. That is, a system that, given a certain input, will always give the same output. These are systems that would take 2 + 2 and output 4. For the first 40 years of AI, from the 1950s until the 1990s, this was the dominant form of AI. Gary Marcus is the most vocal modern proponent of symbolic AI. To give an example in our world it’s a rules-based system that might sort donations into fixed categories based on amount.
Context-aware AI
This is AI that learns patterns and structures from data in order to then generate new, or novel, content based on that data. It is unpredictable. In computer science it’s generally known as Connectionist AI. Depending on the context - say you had asked it for a joke - 2 + 2 might generate something completely different from 4. This is now the dominant form of AI and recently saw Geoffrey Hinton, Yann LeCun, and Yoshua Bengio receive Nobel prizes for work in the field. For charities it might notice patterns in donor behavior and adapt its categorisation based on multiple factors.
From the perspective of charities it’s important to remember that both of these flavours of AI have value. It would appear that context-aware AI might end up being a glue that enables more deterministic systems. That’s something being explored by researchers in the nascent field of neuro-symbolic AI.
It is moving fast
We first released this playbook in February 2025. Three years ago few people knew what GPT3 was. Only a handful of us were working with AI (Edd, one of the authors, was running an AI startup). This was mostly because the capabilities of AI were niche. Rules-based AI would hit complexity ceilings, like a tower of dominoes, where changing one rule would trigger a cascade of unintended effects. Context-aware AI was showing glimmers of value but was often unreliable and would give unpredictable, strange, responses. Emily Bender witheringly called these models stochastic parrots and that seemed valid.
There are many technical reasons why this changed. But perhaps the easiest way to see this is by comparing image generators in 2023 and video generators 18 months later.
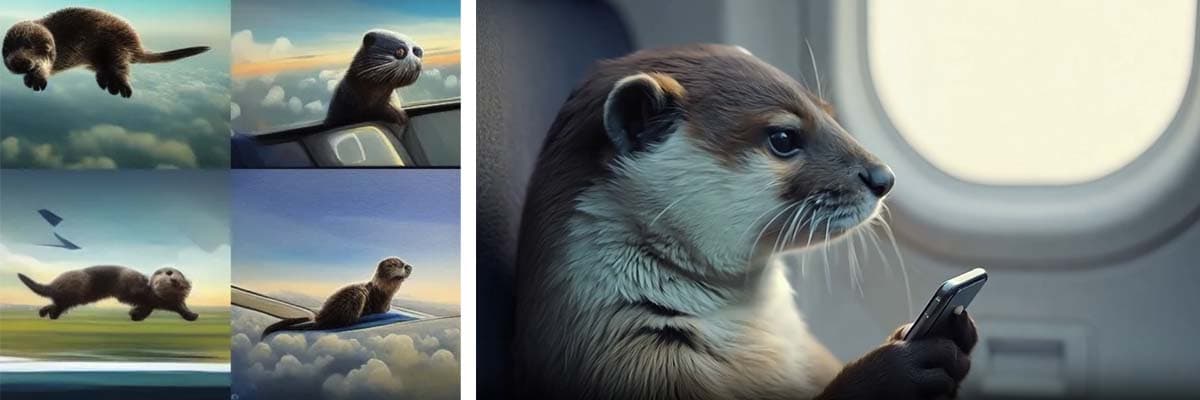
The originals are at One Useful Thing's blog.
The advancements displayed in the above images can be seen across all forms of AI. Pick a discipline, any discipline, and AI has shown remarkable gains in the past few years. In short, AI is becoming faster, cheaper and more capable.
There are three reasons why the technology is developing quickly.
The biggest change has been the impact of exponential growth in computing. Computing power doubles every two years following Moore’s Law. This exponential growth isn’t intuitive for humans but means computers are more than 1,000 times faster than they were in 2000. This has been accelerated even faster by the fact AI can rely on a type of maths called floating-point arithmetic. This is the same maths used by video games to generate 3D graphics and so graphical processing units (GPUs) turned out to be incredibly efficient at processing artificial intelligence.
The second change is money. The amount of money being invested into this space is breathtaking and there are very few historical references that can be made. In 2024 OpenAI had $6.6 billion invested in it, this was the largest ever VC investment. In early 2025 it was announced Softbank, Oracle, Nvidia and the US government would spend $500 billion on AI infrastructure in the coming years. The FT reports Alphabet, Meta, Microsoft and Amazon intend to spend $320bn in 2025 on AI. The closest comparison might be the “Railway Mania” of 1845 - 1847, but even that investment cycle is on a smaller scale compared to investments in AI.
The third change is the ripple effect that ChatGPT created. Within 3 months it had gained 100 million users. This is the fastest growth curve in history. The web took 7 years to reach that size, Facebook almost 5 years. As of writing it’s the 7th most popular website on the internet. ChatGPT showed what was possible even if it only made it 90% of the way there. This has led to many other companies to start competing in the space and for organisations to start investing in the technology.
It is causing societal change
AI is causing massive societal change. To take three examples amongst many.
Changing our employment
AI is creating a form of technological unemployment. Beyond the immediate impact, there’s the fear factor. It’s always easier to see the jobs that will disappear before future, promised, industries arrive. This has been the driving force behind recent redundancies in the tech sector that will continue into the near future. Salesforce, for example, has said they’re not planning to employ any new developers in 2025
It’s always easier to see the jobs that will disappear before future, promised, industries arrive.
Changing our knowledge
AI-powered systems sometimes present partial or incomplete information, particularly on contested topics. For example, the AI tool DeepSeek R1 will refuse to tell you about events like the Tiananmen Square massacre. Similarly, generative AI models like GPT only partially address sensitive subjects such as the Indian Partition. AI has the potential to shape, filter, or even distort public understanding of historical and contemporary issues. We talk more about this in the Bias, misinformation and trust section.
Changing our relationships
AI's role in personal relationships is evolving, with the advent of AI-generated companions influencing human interaction. For example, platforms like Replika and ChatGPT are increasingly being used as digital companions, raising awkward questions about human-AI relationships. Platforms like Meta and X are talking about, or have already, introduced AI avatars who can interact with users on the social network.
There are ethical and environmental concerns
Artificial intelligence embeds complex ethical challenges into our social fabric. As these systems process vast amounts of human-created information, they inevitably reflect and sometimes amplify societal biases. This moves beyond simple technical questions into fundamental concerns about fairness, accountability and transparency.
There is also concern about the environmental cost of the technology. While individual AI interactions might seem negligible, the aggregate impact of these systems is significant. Data centres supporting AI systems now consume substantial portions of national power grids, creating tensions between digital advancement and environmental sustainability. Sasha Luccioni, at HuggingFace, has been speaking on the environmental challenges of AI for a number of years and is worth following in the conversation.
It’s not going away
AI is already becoming deeply embedded in our core infrastructure. From Microsoft 365 to Google Workspace, AI is already woven into the standard tools that charities use daily. OpenAI has announced there are 300 million weekly users of ChatGPT. Google has 400m users of Gemini. Even organisations actively trying to avoid AI are likely using it through their existing software subscriptions and even more likely to have team members already using it for co-intelligence.
This shift is being reinforced by the government. The UK's AI Action Plan, released in January 2025, commits to expanding national computing capacity twentyfold by 2030 and establishing dedicated AI Growth Zones. These aren't temporary measures but permanent structural changes designed to position Britain as an "AI superpower" and the Labour government is making a significant bet that this will create the country’s much needed economic growth. Similar initiatives are emerging globally, with the previously mentioned Stargate project in the US.
The transformation extends beyond technology and policy. The job market is evolving to prioritise AI-related skills, whilst demand is weakening for traditional creative roles as companies hold off hiring. There are regulatory frameworks like the EU AI Act that may create permanent governance structures rather than temporary oversight. The same is true in how copyright is being approached. It seems like governments are going to prioritise the needs of large corporations over those of individual creators in order to enable Large Language Models to have the data they need.
It also means user expectations are shifting. Beneficiaries and donors increasingly expect AI-enhanced services, much as they came to expect mobile-friendly websites in the 2010s. They are used to interacting with Shein, Zara and TikTok. For charities, this creates both urgency and opportunity. Like previous disruptions it’ll be those who adapt thoughtfully who can enhance their impact, while those who delay risk falling behind in their ability to serve beneficiaries effectively.
The momentum will create positive feedback loops for the technology. The cost of implementing AI is following a traditional experience curve - becoming cheaper and more accessible each year. Like websites, email and cloud computing became essential tools for fulfilling a charity’s mission, AI is emerging as a technology to make sense of, and start using.
You need to interact with it
We’ve worked with many leading charities in the UK on interacting with, or integrating with, artificial intelligence. There has been a stark contrast between organisations who want to take a philosophical approach to the subject and those who want to take a practical approach. Our belief is that this is happening because of fear. This is understandable. AI is frightening. All general purpose technologies have created negative consequences at the same time as creating positive ones. The only way to engage with what might be positive or negative about the technology is to engage directly with it. That means investing the time to build the foundations, understand risks + harms to then start enabling team members to work with it.
3 - AI risks + harms
Charities have unique responsibilities when implementing AI given their role in running activities that serve the public interest or common good. We believe AI opportunities are significant - it’s why we’re supporting charities to integrate AI - but getting things wrong could harm beneficiaries or damage hard-earned trust. Understanding potential risks helps organisations innovate responsibly while protecting their mission and those they serve.
The key is to treat AI outputs as suggestions rather than facts. Implement appropriate human oversight, especially for public-facing content, and maintain clear processes for fact-checking and verification.
Bias, misinformation and trust
Large language models have been trained on human created information like CommonCrawl. Some of those are broadly trusted, and trustworthy, sources such as the BBC or academic journals. But many sources, Reddit, Twitter, 4chan etc. are not renowned for reliable information. This makes LLMs vulnerable to generating harmful content, as demonstrated by early versions of GPT models.
People are incorrect, biased and mistaken. AI systems can reflect, and sometimes amplify, these human flaws. The technology has improved significantly since early releases, with better safeguards and more reliable outputs, but the risk hasn't disappeared entirely. For charities, this means being particularly careful when using AI to generate content about sensitive topics or provide information to vulnerable people.
The key is to treat AI outputs as suggestions rather than facts. Implement appropriate human oversight, especially for public-facing content, and maintain clear processes for fact-checking and verification. Remember that AI should enhance rather than replace human judgment.
Cultural impact
AI is already changing how we create and consume information. We can see this with the emerging rise in 'AI slop' - low-quality, mass-produced content that prioritises quantity over quality. This flood of AI-generated material risks drowning out authentic human voices and making it harder to find reliable information.
There are broader societal concerns too. Smartphones have changed how we think and interact but AI could fundamentally alter our cognitive abilities. When tools like ChatGPT can instantly generate answers, we might become less inclined to develop deep understanding or engage in critical thinking. For charities, whose work often involves complex social issues, this could affect how people engage with and understand causes. Use of AI needs to be considered when thinking about opportunities and risks.
There is also the challenge that the copyright issues surrounding AI are so complex that no-one knows what will happen. To try and address this problem all the major players have some form of indemnification against copyright claims (here’s the one from Google). That may solve legal risk but it doesn’t necessarily address the moral status that many feel should be assigned to copyrighted materials.
All of these are societal level issues and even for the largest charities likely outside of their direct scope but are all hard questions that need to be evaluated and integrated into any AI guidelines you produce for your governance.
Environmental impact
Data centres are using massive amounts of electricity. The US Department of Energy released a report in December 2024 showing how total data center electricity usage climbed from 58 TWh in 2014 to 176 TWh in 2023 with estimates of an increase between 325 to 580 TWh by 2028. If it hits the high number it'd be 12% of total US electricity usage. In the Republic of Ireland it's already above that figure with data centres using 21% of electricity in 2023.
Demand for digital services is growing rapidly. According to the IEA between 2010 - 2023 the number of internet users more than doubled across the globe and internet traffic expanded 25-fold. This isn't just about artificial intelligence but factors such as:
- Increase in remote, and hybrid, working (e.g. video calls)
- Increasing on-demand / high resolution streaming (e.g. Netflix)
- Bitcoin and crypto currencies (e.g. the stupidity of trying to brute-force guess a random 10^22 number in order to get a Bitcoin bounty)
- Online gaming (e.g. popularity of Massively Multiplayer Online Role-Playing Games (MMORPGs))
Artificial intelligence clearly is a factor in this but it’s likely not as bad as we think. We can consider this from two directions.
One: the use of AI makes certain tasks faster. If we’re designing a new user interface and it would take 5 days to create without AI, but 1 day to create it with AI then we will have used far less overall energy.
Second: GPUs are incredibly efficient. Our research suggests that each element of value being created by a large language model (a token) requires roughly 16 joules of energy to produce. As an imperfect comparison, an energy efficient car requires between 1500 - 2000 joules in order to move just 1 metre. So, a 1 KM car journey is using as much energy as it takes to generate 62,500 - 125,000 tokens, which is about four or five times more than an average user would need on a daily basis. Another way to look at this is that an average user interacting with a large language model is using the same amount of energy as someone streaming 60 minutes of video. This isn’t nothing but it’s certainly much less significant than we intuitively think.
Hallucinations
One of the most discussed risks with AI is its tendency to 'hallucinate' - to generate plausible-sounding but incorrect information. Current AI models have hallucination rates of around 1 - 2%, which might sound concerning. However, this needs to be put in perspective: humans often perform worse at similar tasks. Professional fact-checkers have error rates at about 4 - 7%, data entry error rates are around 1 - 5%, or to extend it to either an entire document, or spreadsheet, level the chance of human error is almost 10%.
There’s a difference in the type of errors made. Humans typically make 'reasonable' mistakes based on similar experiences or logical connections. We might confuse dates of historical events that happened around the same time or mix up formulas. AI systems, on the other hand, can make errors that would seem obviously wrong to humans - like confidently stating that Paris is a US city or creating impossible historical scenarios.
This distinction is potentially useful. Human errors can be harder to spot because they often seem plausible, while AI hallucinations tend to be more obviously wrong once you look closely. For charities, this means different approaches to verification might be needed: human-generated content might need careful fact-checking against reliable sources, while AI-generated content can often be verified through common sense checks and looking for obviously incorrect statements.
Additionally, there appears to be strategies emerging that enable prompts to be written in a way that can reduce the risks of hallucinations, or at the very least reduce the model's over-confidence in its answer.
Risk of distraction
Implementing AI requires time, resources, and attention that could be spent on other priorities. There's a legitimate concern that focusing on AI might divert energy from core charitable work. However, this isn't a binary choice. The key is finding the right balance: start small, focus on specific problems where AI could genuinely help, and scale based on results.
Risk of inaction
When facing technological change, the risks of action are often more visible than the risks of inaction. We can easily list potential problems with AI implementation, but it's harder to quantify opportunities missed by not engaging. Consider how charities that were slow to adopt digital technologies struggled to reach supporters and deliver services during the COVID-19 pandemic. As AI becomes more embedded in how people work and communicate, organisations that don't develop basic AI capabilities risk falling behind in their ability to serve beneficiaries effectively, engage supporters, and operate efficiently. The goal isn't to chase every new technology, but to maintain the capability to adapt as the landscape changes.
4 - Foundations to understand AI
The foundations to understand AI need to balance considered preparation with active learning. While many organisations feel pressure to adopt AI quickly, the key is finding the right pace - one that brings your team along while maintaining momentum. This section outlines practical steps that help you build understanding while moving forward, combining careful consideration with hands-on experience.
The foundation phase is moving forward purposefully. You'll want to understand your starting position and bring your team along, but you'll learn most by doing. The activities in this section help you create that balance, setting up guardrails that enable experimentation while managing risks.
Many organisations feel pressure to adopt AI quickly, especially as they see others in the sector moving forward. However, rushing into implementation without working with your team can lead to resistance, wasted resources and missed opportunities.
Outside support
We should acknowledge our conflict of interest here - being an external agency who works with charities to understand AI - but we think it’s incredibly useful to have some outside support when considering the foundations of AI.
There are three reasons why it’s helpful to have outside support.
- External facilitators, and collaborators, can see and hear things that folks internally might not be able to, even with exactly the same skills
- AI is a new field and it’s highly unlikely that most charities will have the necessary expertise in-house
- AI is a field that seems unlikely to ever become a full-time in-house capability. This is different from the internet, which was the last big technological change charities went through, where digital skills could obviously be embedded within the Marketing + Communications teams
Make Sense Of It, the agency that we founded, would be super happy to support. But we’re not the only agency that’s able to help. You might find value with CAST, Modern Change, The Social Change Agency, Torchbox, The Social Innovation Partnership, Nesta, MantisNLP, IDEO, &Us to name just a few.
Many funders and foundations are offering support for exploring AI to charities. There is a sector-wide recognition of just how hard this challenge is. If you’re worried about the cost of bringing in outside support it would be worth seeing who are offering small grants in the space.
To help benchmark costs our feeling is that much of the work for the foundations can be done for between £5k - 20k depending on the scale and number of people who need to be involved. Reviewing our own proposals the median is £8k.
Mapping threats and opportunities
AI is going to have ripple effects.
An example:
Working with a charity whose main mission is to disseminate information it was clear that generative AI represents an existential crisis. If someone could simply get the information from ChatGPT, why should they interact with the charity? But, at the same time AI gives them an opportunity to massively increase their reach by connecting research together, by allowing people to choose the complexity of language or by being able to more quickly make sense of the world and express their point of view.
A map helps you prioritise what’s important.
There are many ways that you can start mapping threats and opportunities but this is the most straightforward way that we’ve found that allows the most people to contribute:
- Map the work that your charity is doing
- Map the beneficiaries, supporters and advocates of your charity and how you’re interacting with them
- Map your income streams and donor journeys
- Consider both immediate fundraising opportunities and longer-term threats
- List all the possible positive opportunities that AI could create in order to augment the work or improve relationships (e.g. personalised donor communications)
- Do the same for the negative threats that AI could create (e.g. no longer being able to reach donors through traditional channels)
- Once you have your lists get them into priority order through voting
If you’re familiar with systems design then the above will sound familiar to you as a way to map your eco-system. If you wanted to run this session yourself there’s quite a useful Miro template here.
Governance + compliance
You won’t be able to make progress with integrating AI without exec buy-in. To do that you’re going to need to establish basic governance before starting to use AI. We say ‘basic’ because AI is developing too quickly to enable the creation of a perfect framework. You'll learn more through practical experience than theoretical planning.
We’ve seen the governance + compliance work sit with various people in different organisations. The Chief Information Officer (CIO) seems to be the most popular choice for this work, but we’ve seen it sit with the CEO, Comms Director, Director of Digital and Head of Transformation. Where it’s worked best is when it’s treated as a convening role where whoever is in charge is responsible for bringing people together rather than creating the governance themselves.
You'll learn more through practical experience than theoretical planning.
Start by engaging your board and trustees. They need to understand both the opportunities and risks to make informed decisions about resource allocation. Identify an executive sponsor who can champion AI initiatives and help navigate organisational barriers. Set up quarterly reviews with key stakeholders to assess progress and adjust course as you learn.
It is useful to create a simple AI ethics framework that can evolve. Focus on basic principles that protect beneficiaries while enabling exploration. Ideally these principles should be a mixture of bottom-up and top-down priorities. We often run what we call an AI Fundamentals workshop where we capture the concerns and fears of team members to build these AI Principles.
The purpose of this is to create some ‘guardrails’ about what types of AI usage are and aren't acceptable for your charity at this stage. There’s value in establishing basic reporting processes that focus on mission impact rather than technical metrics.
Remember that your governance approach will need to evolve as your understanding of AI grows and as the technology itself develops. Regular reviews help ensure your frameworks remain appropriate while maintaining focus on your charitable objectives.
Managing pace of change
You need to get staff buy-in. Managing change around AI requires a different approach from previous technology transformations. The pace of AI development means organisations can't rely on traditional change management methods that assume slow, controlled transitions. Instead, charities need to balance rapid technological advancement with thoughtful implementation that protects their mission and values.
Start by understanding where you are. Your staff are likely already using AI tools, whether officially sanctioned or not. Rather than trying to control or prevent this experimentation, use it as valuable insight into where AI could add value to your organisation. Run a baseline survey to understand current attitudes and identify both enthusiasts and those with concerns. You can see a survey example here. You could follow this with stakeholder conversations that dig deeper into hopes and fears around AI adoption. Here an external facilitator, or at least a newer team member, can be helpful so people feel free to share their opinions.
It’s important to create a positive-error culture around AI. Arguably it’s important for everything, but we’re just focussed on AI! Best practices for AI are still emerging, and it’ll require experimentation and learning to get this right for your charity. Staff need to feel safe trying new approaches and reporting when things don't work as expected. This openness helps your organisation learn collectively while identifying potential risks early. The goal isn't to eliminate all mistakes but to learn from them quickly and adjust your approach accordingly.
Staff need to feel safe trying new approaches and reporting when things don't work as expected. This openness helps your organisation learn collectively while identifying potential risks early.
Being transparent with beneficiaries, supporters and advocates
Being open about your AI journey is essential. Your beneficiaries, supporters and advocates need to understand how and why you're using AI. This isn't about making grand statements but rather about clear, honest communication about where AI is supporting your work. Share both successes and challenges, and be explicit about safeguards you've put in place to protect sensitive information. RNIB have done this well, such as this blog looking at the potential of AI and this blog talking about their partnership with Microsoft.
Create channels for feedback and be ready to address concerns. If you're using AI in service delivery, make sure people understand when they're interacting with AI systems and what human oversight exists. This transparency helps maintain trust while demonstrating your commitment to responsible innovation.
Resourcing + timelines
We are asked about resourcing and timelines frequently. There isn’t a one-size-fits rule.
Resourcing
Some smaller charities have involved everybody, others have only involved senior leadership, whilst others have aimed to get a spread of junior and senior voices. We feel the latter approach is likely to be the most resilient approach enabling a diversity of opinions and creating trust through the organisation. One of the people involved should be on the senior management team, or at the very least, frequently reporting back to the SMT. That said, if a member of the SMT isn’t resourced into any of the work it sends a fairly clear signal about priorities to the rest of the team.
Timelines
Again timelines have ranged dramatically from charities we’ve worked with. The most successful processes have been reasonably quick though. It’s entirely possible to understand initial usage, run workshops and some lightweight training in 6 weeks. It gives a sense of purpose and momentum that can carry on beyond the phase. By contrast Edd was involved in a project that lasted almost a year. By the time they finished it was time to restart because everything had moved on so much.
Success at this stage isn't about having perfect plans or comprehensive frameworks. Instead, focus on building understanding, establishing basic governance, and creating momentum for thoughtful AI adoption. The goal is to get in place lightweight documents that enable you to go from theoretical discussions to practical exploration while maintaining appropriate safeguards for your mission and beneficiaries.
Part two: how charities can use AI
There are three ways that charities can start using AI. Each of them has slightly different implications that we explore.
Augmentation enables members of your team to access AI systems so they can use them as a form of co-intelligence.
Integration places AI directly into your processes through automation or by directly interacting with an AI system.
Finally AI outsourcing is where you take advantage of new types of agencies that are emerging who are committed to taking an AI-first approach to increase value and reduce costs.
5 - AI augmentation
Augmentation, often called co-intelligence, is about using artificial intelligence, and particularly generative AI, to enable team members to do more than they previously could do.
When former world chess champion Garry Kasparov lost to IBM's Deep Blue in 1997, he decided to team up with AI. He created the concept of 'Advanced Chess', where humans and computers work together. Humans brought strategic thinking and creativity, while computers handled tactical calculations. This created gameplay stronger than either humans or machines at the time could achieve alone.
The same principle applies to how charities can work with AI today. Generative AI excels at processing information, spotting patterns, and handling routine tasks, while humans bring mission focus, ethical judgment, and deep understanding of beneficiary needs. By thoughtfully combining these capabilities - what is called Co-intelligence - charities can achieve better outcomes than either could alone. This is particularly important in a sector where under-resourcing is often a barrier to impact.
Note: since co-intelligence is now so entangled with generative AI this section is almost exclusively about generative AI.
Augmenting human capabilities
AI augments rather than replaces human capabilities. Every AI interaction should begin with human intent and end with human judgment, creating a continuous loop of purposeful engagement. There should always be a human-in-the-loop.
This approach means designing workflows where AI supports rather than directs decision-making. For instance, when using AI to assess grant applications, the system might help identify promising candidates, but the final decision always requires human evaluation considering factors like community impact and alignment with your charitable objectives.
Think of AI as a particularly capable assistant, one that can process information quickly and spot patterns, but that needs clear direction and oversight. One charity we work with keeps referring to it as their over-enthusiastic graduate analyst. Your team members bring crucial elements that AI cannot replicate: empathy, contextual understanding, and alignment with your charitable mission.
Creating effective human-machine interaction involves:
- Setting clear boundaries for AI use
- Establishing checkpoints for human review
- Building staff confidence through transparent processes
- Documenting decision-making rationale
- Maintaining focus on beneficiary outcomes
You’re not trying to automate anything here. The team, the people, will still be involved. It’s the interaction between the human-machine interaction that really creates the value!
Prompting
Prompting is the process of instructing an artificial intelligence system in a way that will create value. It’s now most commonly used to describe how we interact with AI chat interfaces like Anthropic’s Claude or OpenAI’s ChatGPT.
Prompting is important and it’s likely to continue to be important for at least the next few years. Through the work we’ve done training teams we’ve seen first hand the massive difference “good” prompting has compared to poor prompting. However, the goal isn't to craft the perfect prompt but is to use AI systems interactively and iteratively.
Effective prompting requires providing both context and constraints. Rather than simply asking "Write me a fundraising email", you might specify "Draft a fundraising email for our regular donors, focusing on our recent youth mental health programme successes, maintaining our warm but professional tone of voice, and keeping it under 300 words."
Specificity helps the large language model understand both what you want and how you want it delivered. You can think of it like briefing a new team member, the more context they have the better they can meet your needs.
You'll get better at prompting through practice. Start with simple requests and gradually increase complexity as you learn how the system responds. Pay attention to what works and what doesn't, and build on successful approaches. This is the case for both big and small tasks. But as a starting point breaking complex tasks into smaller steps will almost always get you better results than trying to accomplish everything in a single prompt.
If you want more information on this a useful website for some principles around prompting is at the Art of the AI Prompt. Anthropic has a useful guide on best practices. Ethan Mollick also has a very accessible blog post on it here. And, to, briefly, mention this is also a training course we can run for you.
Data safety
You should pay for the tools your charity is using. If you’re not paying, as we’ve learnt with Facebook et al, it means that you’re the lunch! The free tiers of services from OpenAI, Anthropic, Google and Microsoft all have provision for them to use your data for future enhancements to their models. If you pay as an individual you can opt out of this, and if you’re on either a team or enterprise tier then you’re never included. Requests via their APIs are also excluded from data collection.
You should pay for the tools your charity is using. If you’re not paying, as we’ve learnt with Facebook et al, it means that you’re the lunch!
This is one of the reasons that it’s important to run through the Foundations process in order to understand who in the team is already using AI. There is a high chance that they’re not paying for the service they’re using, which means that the data they’re sharing - organisational data - is being used to train models.
This is an issue that all of the major players are aware of. It is the biggest barrier for onboarding customers for them. This was made worse by the fact early in ChatGPTs history there was a bug that revealed customer conversations. You can read OpenAI’s Security and Privacy documents here, which match, more or less, those of Google, Microsoft and Anthropic.
Data safety is also an area where - if you have the budget - there may be value in exploring local, open-source, solutions like DeepSeek or Llama 3. You can run these models on a service like HuggingFace and enable team members to access it. But, to achieve something close to comparable to a SaaS solution you’ll need to spend at least $1,000 / month. A lower cost solution, if your team’s computers are powerful enough, is to use a service like Ollama, which will let your team run models locally.
We’d like to stress that data safety is relatively low risk with these models. It feels somewhat analogous to how we needed to be aware of how we were interacting with Google in the early 2010s. The service was clearly taking advantage of our data - if we weren’t paying for the service - but wasn’t reproducing verbatim that data. If you put your in-process Annual Review into a chatbot it won’t be that another charity can extract it.
Procurement and accessing tools
Getting AI tools properly set up in your charity requires coordination across teams. Your legal team reviews terms of service and data protection. IT assesses security and technical needs. Finance handles budgets and payment terms. Programme staff explain what they need the tools to do.
Create a simple process for staff to request tools. This could be a form that gathers key information: what they need, why they need it, and how many people will use it. This helps your teams evaluate requests quickly.
When approving tools, start small. Get a few licenses, perhaps test with a specific team, then expand if it works well. This lets you learn what support people need while managing costs. We recognise this might be challenging for the IT team who tend to procure large-scale licenses.
Set clear guidelines about who can access which tools and what they can use them for. Regular reviews help ensure tools remain useful and cost effective.
Remember that making it easy to access approved tools is the best way to avoid people using unsafe alternatives and creating shadow IT. From our experience this might require an advocate in SMT directly pushing for these tools to be made available.
Measuring ROI on team use
As we mentioned within the data safety section you should be paying for your tools. The standard cost is £20 / month. This has proven to be a significant barrier for charities who perceive it as an additional cost. We’d suggest tracking the ROI might demonstrate that it’s actually very good value for money.
The simplest way to calculate ROI is around time saved.
Someone on an annual salary of £45,000 has an hourly salary of about £22. For your monthly subscription to break even they only need to save about 15 minutes / week. The higher the salary the less time saved is required.
We have a simple calculator here to help you calculate this. From our experience, assuming people are using the tool, the subscriptions pay for themselves very quickly!
We’d note time saved isn’t the best measure though it’s certainly the easiest. With co-intelligence you’re able to expand your team’s capabilities and do things that weren’t previously possible. This sort of value added calculation is more complex but valuable to spend the time doing since it’s highly likely that it’d make these tools even more obviously valuable.
For teams focused on income generation, ROI calculations should consider potential gains alongside time saved. For example, if AI-powered analysis helps identify just one major giving prospect or helps secure one additional grant per month, the return could be orders of magnitude higher than the tool costs. Even small improvements in supporter retention or average gift amounts, multiplied across an entire base, can create significant returns on AI investment.
Measuring energy use by your team
We’re currently working on research around large language energy use. We know that concerns around energy use - and how it needs to be included within impact reporting - is a barrier for charities when using these tools.
Our current estimate is that each unit of value from a large language model - a token - takes about 16 joules of energy to produce.
From our own work, and observations of what people in charities are already doing, we think the average person is having roughly 5 conversations per day, with roughly 10 messages per conversation and that correlates to about 100 input tokens per message and 500 output tokens per response. That equates to about 500,000 joules (0.5MJ). For context this is the energy contained in about 15ml of petrol, or enough to move a car about 200 metres.
You can also measure the maximum the team is likely to be able to use. All the major players have similar limits. Anthropic’s Claude’s limits are documented here. But in brief you can send ~150,000 tokens every 5 hours. Assuming Claude responds with the same number of tokens that would mean 2,400,000 joules (or 2.4 MJ) of energy. For context this is about the energy in 75 ml of petrol, or enough to move a car about 1km. Not nothing, but as a maximum possible energy output not massive.
To frame this a slightly different way. A charity’s office of 10 people is about 100 m². That will require roughly 40,000 lumens of light. Thanks to LEDs we can now get ~100 lumens / watt. Over 8 hours this will be roughly 12 ,000,000 joules (12MJ) of energy. If all 10 people in the office use a large language model to our average then they’ll have used 5MJ of energy. That is, the office lighting accounts for double the energy usage compared to the use of AI.
Upskilling your team
Your team won’t know how to use generative AI innately. There are some who will find it easier than others. It seems to be generalists, people with a small amount of knowledge about many things, who get the most value. Humanities students - or at least folks with good ability to express their point-of-view - also seem to have an advantage. This is an interesting inversion of who previously got most value out of computers. It has traditionally been people with narrow skills and the ability to use highly specialised computer language syntax that have been able to get the most value from machines.
Your team will need upskilling to take advantage of this change.
You can enable upskilling organically. That means giving people time and space to explore tools, make mistakes and learn from them. It likely means creating a community of practice (or similar) so that people can share their knowledge through the charity. These informal settings often spark innovation as people share what works for them.
For more structured support, consider professional training. While this playbook's authors offer training services, there are also other providers, online courses, and workshops available. The key is finding training that fits your charity's specific needs and culture.
Whatever approach you choose, remember that upskilling isn't a one-time exercise. Build time for learning and exploration into your regular workflows. Investing in your team's capabilities will increase in value as AI continues to evolve and you’re able to respond more quickly.
6 - AI integration
AI enables charities to automate more of their services than was previously possible. Where automation once meant simple rule-based chatbots or basic form processing there’s now the potential for sophisticated service delivery that can potentially work around the clock, handle complex cases, and scale to meet demand.
Here, AI is broader than just generative AI. There are some services that would greatly benefit from some rule-based AI systems, others where generative AI is a perfect solution, and another category where a combination of the two works well.
The cost of automation
Implementing AI carries both financial and social costs that charities need to consider. The direct costs are straightforward: the cost of the project, plus time for migration and onboarding. While these costs should pay for themselves through efficiency gains, they still need to be budgeted and justified.
Less obvious might be the cost to your culture. Many charities, particularly those that are volunteer-led, create value through networks of people working together to support their mission. A foodbank's weekly sorting session, for instance, doesn't just distribute food but also creates community connections and gives volunteers meaningful ways to contribute. Automating these processes might improve efficiency but could reduce valuable human interaction and intangible benefits
Automation, of course, is not an all-or-nothing choice. Charities can, and should, use AI to handle routine tasks while preserving human interaction for complex situations.
There are also implications for beneficiaries who rely on personal contact. While younger service users almost certainly prefer digital interactions others may feel excluded or unsupported by automated services. Some may simply need the sense of understanding that comes from human connection.
Automation, of course, is not an all-or-nothing choice. Charities can, and should, use AI to handle routine tasks while preserving human interaction for complex situations. There’s obviously a challenge feeling out where that line is, and trying to define that grey area is essential to using AI in any service.
The need to scale
AI enables scaling in ways that previously weren't possible.
Without finding ways to scale their services charities risk making their support increasingly costly and reaching fewer people, even as need grows.
Charities who rely on manual processes are creating something akin to Baumol's Cost Disease. This effect was named after William Baumol who showed that it still takes exactly the same number of musicians the same amount of time to perform a quartet as it did in Mozart's era. But - thanks to productivity gains elsewhere in the economy through automation and computing - musicians’ wages have had to increase in line with the economy. This has made classical performances increasingly expensive and exclusive.
Without finding ways to scale their services charities risk making their support increasingly costly and reaching fewer people, even as need grows. For some challenges, such as trained nurses who could respond to people going through treatment, there simply aren’t enough experts to scale the service even if money were no object. AI offers a way to get past this problem.
A good example of a charity achieving this is Girl Effect and their app Big Sis. In 2022 it was able to reach 75,000 young women who had over 1 million conversations. This scale would have been impossible to achieve through traditional staffing models.
The challenge for charities is finding the right balance - using AI to extend reach while maintaining quality and personal connection where it matters most. This might mean automating initial contact or routine queries while preserving human interaction for complex cases or crucial moments in someone's support journey.
We can see the same tension with fundraising. Charities need to appeal to multiple audience segments simultaneously. That means maintaining strong relationships with long-standing supporters while developing new approaches to engage future generations of donors. Resource scarcity has often forced charities to focus on a single audience at a time creating a perpetual tension between tradition and innovation. AI should enable charities to more easily scale these conversations whilst allowing them to spend more quality time with their most important donors.
Scarcity to abundance mindset
AI fundamentally changes what's possible for charities by removing traditional resource constraints. This happens in two ways. Rules-based AI can process thousands of cases consistently and instantly - whether that's matching donors to projects or assessing support applications. Meanwhile, generative AI can produce multiple creative solutions for tasks like drafting communications or designing campaign approaches.
This shift requires rethinking how we approach tasks. No longer limited by processing capacity and resource we can explore numerous possibilities. We can do this both through systematic assessment of large datasets and through creative exploration of different approaches. It hopefully means the end of first-solution thinking!
Abundance creates new challenges. Having 500 possible - plausible - solutions, or being able to process every case instantly, isn't helpful without good systems for managing and evaluating outputs. A skill that we expect to emerge is how best to structure processes and assess results effectively. This is not something they taught with Prince2! Charities need to develop frameworks for managing this shift from scarcity to abundance while maintaining focus on their mission.
Talking in data
Structured data, think spreadsheets and well-curated databases, has obvious value. But charities often have vast amounts of unstructured data in case notes, meeting minutes, emails, and beneficiary feedback that traditionally was too complex to analyse systematically.
AI changes this equation. Generative AI can help make sense of unstructured information, finding patterns and insights that would be impractical to discover manually. These insights can then feed into more traditional rule-based systems for consistent decision-making.
Even data you didn't know you had becomes valuable. Every interaction, email exchange, and support conversation potentially contains patterns that could improve service delivery. The challenge isn't just gathering data anymore, it's identifying which information could be valuable and finding ethical ways to use it.
The challenge isn't just gathering data anymore, it's identifying which information could be valuable and finding ethical ways to use it.
One of the key challenges we see for charities in 2025 and 2026 will be to get on top of their data and start using it effectively.
Service integrations
In our conversations with charity leaders we’re often asked, “OK, but what does an AI service actually look like…”, and here are some answers:
Matching services
AI excels at connecting needs with resources across your charity. Whether matching volunteers to opportunities, donors to projects or beneficiaries to services, AI can process multiple factors simultaneously to create better matches than manual methods. For animal charities, this might mean matching pets to potential homes based on lifestyle, experience and living situation. For volunteer organisations, it could mean connecting skilled volunteers to projects that need their expertise.
For fundraising teams, this means better matching of supporters to specific projects or appeals based on their interests, giving history and engagement patterns. AI can also help match corporate partners to appropriate opportunities or trusts to relevant projects.
Early warning services
AI can spot patterns that indicate developing problems, enabling earlier intervention. This might include identifying signs of mental health crisis from support messages, recognising when families might be approaching food poverty from changing service usage, or detecting when elderly beneficiaries might need additional support based on subtle changes in their communication patterns. These insights help charities provide support before situations become critical.
Knowledge amplification
Many charities have experts whose knowledge could benefit more people if it could be shared more widely. AI can help capture and distribute this expertise. For example, a mental health charity could use AI to help helpline volunteers access relevant clinical knowledge during calls, or an environmental charity could help local groups access specialist conservation advice. This amplifies your experts' impact without requiring more of their time.
Case management support
AI can help staff manage larger caseloads more effectively by prioritising cases, suggesting next steps and highlighting important information from case notes. This is particularly valuable for helping junior staff work more independently while maintaining service quality. The AI acts as a knowledgeable assistant, helping spot patterns and suggesting actions while leaving decisions to human judgment.
Service navigation
Many beneficiaries struggle to find the right support in complex service landscapes. AI can guide people through your services, helping them understand what support is available and how to access it. This might mean an intelligent chatbot that helps people find relevant services, or a system that proactively suggests additional support based on someone's situation. The goal is making your services more accessible without creating new barriers.
Income and engagement integrations
Beyond services AI is transforming how organisations engage supporters and secure sustainable funding. This is particularly relevant as younger supporters expect more personalised interactions and traditional channels face increasing competition for attention.
Key opportunities include:
Supporter journey optimisation
AI can analyse behaviour patterns to identify optimal engagement timing and channels, optimise ask amounts, and predict which supporters might be ready to increase their giving. This helps charities make more effective use of limited resources.
Legacy programmes
AI tools can support the sensitive work of legacy giving by helping identify potential legators through pattern recognition in supporter histories and engagement data, then supporting personalised nurture programmes.
Trust and Foundation research
AI can accelerate application processes by analysing success patterns in previous proposals, suggesting improvements, and helping match projects to potential funders. Whilst human expertise remains crucial for final applications, AI can handle much of the initial research and drafting.
Partnership development
By analysing corporate social responsibility trends and company data, AI can help identify promising partnership opportunities and suggest alignment between corporate goals and charitable missions.
7 - AI outsourcing
Most charities will engage with AI primarily through their external partners and providers. From digital agencies to accountants, your suppliers should be using AI to deliver better value. This chapter explores how to ensure you're getting the benefits of AI from your partnerships while managing any associated risks.
Working with providers
Your providers should be using AI.
Two years after ChatGPT's release, your external providers should have clear policies and practices around AI use. If they don't, it raises questions about their ability to deliver value in a rapidly evolving landscape. The challenge here relates back to the Baumol Effect that we talked about in “The need to scale” section. Just as you wouldn't work with a provider who hadn't adapted to cloud computing or mobile devices, providers without AI capabilities risk becoming increasingly expensive whilst delivering less value.
This isn't about providers using AI for its own sake. Rather, it's about ensuring they're passing on the efficiencies and capabilities that AI enables. A digital agency using AI in their design and development process should be able to deliver projects more quickly and at lower cost. An accounting firm using AI should provide deeper insights from your financial data. A fundraising consultant should be leveraging AI to develop more effective strategies.
When engaging with providers, ask about:
- Their AI policy and how they ensure responsible use
- Specific ways they're using AI to improve their service
- How AI-driven efficiencies translate into better value for you
- Their approach to data protection when using AI tools
- How they maintain quality while using AI
The goal isn't to demand providers use specific AI tools, but to ensure they're thoughtfully incorporating AI in ways that benefit your charity. This might mean faster delivery times, reduced costs, or enhanced capabilities - ideally, all three.
The goal isn't to demand providers use specific AI tools, but to ensure they're thoughtfully incorporating AI in ways that benefit your charity.
Taking advantage of standardisation
Part of how all forms of artificial intelligence works is through standardisation. The acceleration of digital and design projects is particularly noticeable when it comes to generative AI. All forms of successful innovation have taken advantage of standardisation to help make it easier, or cheaper, to do something, which lets the innovation become more widespread.
Charities need to be aware of this when commissioning work in order to get value. This may mean moving away from processes, or products, a charity was previously close to. You should now consider carefully whether bespoke solutions truly add value or might actually slow you down. It’s normally beyond the scope of charity leaders to care about the “how” of some of their systems but we think there’s value of getting into the weeds in certain areas.
Digital development
The modern web development stack of React, utility classes like Tailwind CSS and component libraries like shadcn/ui creates a standardised environment where AI tools excel. Agencies using these tools can move faster and deliver more value, as AI understands these common patterns and can accelerate development. A charity might prefer a completely custom solution, but this could mean missing out on the accelerating benefits that AI brings to standardised approaches.
Financial services
Standard accounting principles and reporting frameworks enable AI to process financial information more effectively. Providers using standardised approaches can offer deeper insights and faster processing. This is particularly relevant for charity accounting, where frameworks like Statement of Recommended Practice (SORP) create common patterns that AI can learn from and work with.
Data management
Common data formats and structures make it easier for AI to process and analyse information. When your CRM provider uses standard approaches to data organisation, their AI tools can offer better insights and automation. This standardisation also makes it easier to switch providers or integrate new tools in the future.
Monitoring and evaluation
Standardised impact measurement frameworks, like the Social Value Framework, enable AI to compare and analyse outcomes more effectively. Providers using these standards can offer better insights into your impact and more meaningful comparisons across the sector.
Process management
Standard project management methodologies and business processes create patterns that AI can understand and support. Whether it's Agile development or standardised grant application processes, these common approaches enable AI to provide better assistance and automation. No-one cares what process management approach you use, they care about the fact it has been combined in interesting ways in order to create value for them.
Charities are often working on complex, wicked problems, at the edge of society. We know there is a tension here between standardisation and unnecessary reductionism. That being said, we think charities need to seriously consider how much value a bespoke approach may give them if it means they have to move more slowly. Speed of iteration, that is how quickly an organisation can evolve, has always tended to be the most successful form of resilience over perfect plans.
Accelerating digital
We want to reinforce just how much artificial intelligence can accelerate digital. For the last 15 years there has been a move to delivering traditional services digitally. Reach more people, create more time to focus on harder cases.
Where charities once faced months of development work, AI can now help teams deliver in weeks or even days. This acceleration comes from AI handling routine coding tasks, suggesting solutions and spotting potential problems early.
The impact is amplified by modern digital infrastructure. Platforms like Vercel for hosting, Neon Postgres for databases and Firestore for real time data management take care of complex backend operations automatically. When combined with AI, these services let small teams deliver capabilities that once needed entire departments.
This shift particularly benefits charities. A team can now quickly build and test new digital services without large upfront investment. AI helps with everything from initial user research through to deployment and monitoring. Features that once required custom development can often be assembled from existing components, with AI helping to connect and configure them.
Success now comes from understanding how to combine these tools effectively rather than building everything from scratch. Charities should look for partners who know how to leverage AI and modern platforms to deliver value quickly. The goal isn't just faster delivery but creating digital services that can evolve as needs change.
Workshops, ideation and sensemaking
Workshops are a way to convene and align. AI now makes these sessions more valuable at every stage. Before workshops, AI helps facilitators prepare materials and research relevant case studies. During sessions, it can suggest activities and provide real time prompts that keep conversations flowing.
We've found that the most significant value often comes after workshops end. Where facilitators once captured ideas on post it notes or in Miro boards that rarely saw further use, AI can now transform these raw materials into structured insights. Photos of workshop walls, recordings of conversations and digital whiteboard exports can all become properly documented outcomes. AI can spot patterns across multiple workshops, connect related ideas and suggest next steps.
This means workshops no longer need to be isolated events. They can feed into ongoing work, with AI helping to maintain momentum by turning general discussions into specific actions. For charities working with external facilitators, this creates lasting value beyond the workshop itself. An example of this sort of documentation giving a clear overview of a workshop can be found here.
The key is finding the right balance. AI is not going to replace human creativity and quite a lot of facilitation skill is required to understand how AI can best be used. However, good facilitators can now use AI to handle routine documentation and basic analysis, freeing them to focus on group dynamics and deeper insight. This combination of human wisdom and AI capability helps charities get more value from their workshop investment.
Marketing and creative
AI can do lots of the heavy lifting when it comes to creative direction. From interpreting briefs and generating initial concepts to visualising different directions, AI now handles much of the groundwork that previously consumed creative teams' time. This augments creative thinking by enabling designers to see different angles that they can explore. The focus can be on refinement and strategic thinking rather than starting from scratch.
The technology particularly excels at rapid iteration. Rather than presenting two or three concept directions, creative teams can now explore dozens of approaches quickly, identifying promising directions that humans might have missed. AI can generate variations of layouts, explore different colour palettes, and suggest alternative visual hierarchies in minutes rather than days.
For charities, this means creative agencies should be delivering more value. In short, if your creative agency isn’t actively using AI to help with creative then it would be worth asking some hard questions.
Where we don’t think AI should be at the moment is in the final product. There have been a handful of campaigns that have used AI really effectively to generate images - in particular WWF and Breast Cancer Now - but our general feeling is that, for most charities, AI is not the right solution for final production images.
For most charities, AI works best as a tool for exploration and development, with human creators crafting the final assets that represent your brand.
Fundraising
In the commercial world data-driven marketing has been a subcategory of the profession since the 1980s. Since the 2010s it has exploded. Some charities have been taking advantage of this for some time, such as Charity:Water doing multivariate testing on their Facebook ads, but many are doing only very light touch testing ahead of launch.
AI, both rules-based and context-aware, makes testing much more accessible and should be something that is embedded into all campaign thinking. We think there are three particularly interesting areas to explore here.
Synthetic audiences
AI can create detailed models of different audience segments, helping you understand how various groups might respond to campaigns before launch. These synthetic audiences, built from existing data patterns, let you test messaging and approaches more effectively than traditional focus groups or surveys.
Probabilistic modelling
Rather than making single predictions, AI can model multiple possible outcomes and their likelihood. This helps charities understand not just what might work best, but how different approaches might perform across various scenarios, enabling more nuanced campaign planning.
Ongoing multivariate testing
AI makes it possible to continuously test and refine multiple elements of your campaigns simultaneously. Rather than simple A/B testing, you can analyze how different combinations of messages, images, and timings work together to improve engagement.
Fundraising and outreach is not only changing because of AI. The consolidation of digital channels over the last decade means fewer, more standardised platforms where content is distributed. This creates clear patterns of how content spreads and gains attention. Success increasingly follows power law distributions, where a small number of pieces of content capture most of the attention, meaning that, more than ever, it’s important to find social waves that will help distribute your thinking. AI helps you understand and adapt to these patterns, identifying trending topics and optimal timing for maximum reach.
Success increasingly follows power law distributions, where a small number of pieces of content capture most of the attention, meaning that, more than ever, it’s important to find social waves that will help distribute your thinking.
Culture is responding to the change in digital channels, and data-driven campaigns, in interesting ways. As we were rewriting this, in January 2025, Cute Winter Boots started to trend on TikTok. It had nothing to do with clothing but was rather a way to organise resistance to US Immigration and Customs Enforcement's (ICE) deportation raids without being flagged. This is a form of algospeak and designed to confuse AI moderation and obfuscate language. This isn’t a new concept, dialects have always been created to exclude as much as include from conversations. But, when thinking about communicating, and understanding your audience you’ll need to be aware at how quickly culture will shift alongside AI.
Avoiding snake-oil
There are many products on the market that are promising the moon. The old adage of, “If it sounds too good to be true…” still holds in the world of AI. Maybe in a couple of years - if something close to AGI arrives - then we’ll be in a different era of abundance, but we’re not there yet. What we’ve discussed above is about accelerating known, existing, processes rather than creating magic.
This creates a tension for charities. You likely have trusted partners who understand your mission and values, but they might be slow to adapt to AI capabilities. Meanwhile, new agencies promising AI expertise might lack deep understanding of the charity sector. The most valuable partners now are often individuals, or small teams, with experience of charities who have broken away from established agencies to focus specifically on applying AI within the context of charities.
When considering partners, look for those who can demonstrate practical applications rather than theoretical possibilities. They should be able to show specific examples of how AI has improved efficiency or impact in similar organisations. Be particularly wary of promises about AI completely automating complex processes or eliminating the need for human oversight.
The best partners will be honest about both the possibilities and limitations of AI, focusing on practical improvements rather than revolutionary change. They'll help you build on your existing strengths while thoughtfully incorporating new capabilities.
8 - Final thoughts
This isn’t traditional charity transformation. It’s not a project that can take 3 years and be neatly planned. The world is moving too quickly.
Success with AI is going to come through practical learning rather than perfect planning... The key is to pilot things, learn from the results, and build on what works rather than attempting to plan every detail in advance.
AI is moving fast. Far faster than anything the authors have experienced in their career! It - for better or worse - demands a different approach from how charities handled previous technological changes.
Success with AI is going to come through practical learning rather than perfect planning. Throughout this playbook, we've shown various ways to start engaging with AI, from enhancing individual capabilities through co-intelligence to integrating AI into services or working with providers. Each offers opportunities for quick, relatively small-scale, experimentation. The key is to pilot things, learn from the results, and build on what works rather than attempting to plan every detail in advance.
This represents a significant cultural shift for many charities. Rather than trying to build perfect, bespoke solutions, the focus should be on combining existing, standardised, pieces in novel ways.
Standardisation and modular approaches aren't limitations but enablers, creating foundations that can be quickly adapted as needs change and capabilities grow. Innovation now comes from how we combine and apply existing tools rather than building everything from scratch.
This modular approach also changes how we manage risk. Instead of betting everything on large transformation projects, charities can run small experiments that limit potential downsides while enabling learning. When something doesn't work, the impact is contained and the lessons can inform future efforts. This makes it easier to adapt and change direction as you learn what works best for your charity and beneficiaries.
The real challenge for charity leaders might not be technological but instead mindset. Success comes from empowering teams to experiment thoughtfully rather than trying to control every aspect of AI adoption. This means building something big from small pieces, moving forward through practical experience rather than theoretical planning. The future belongs to charities that can learn and adapt quickly, not those who spend years planning for a future that will have changed by the time they get there.
About the authors
Edd Baldry is an award-winning designer and innovation leader with two decades of experience helping organisations create positive social change through technology. They've been working on AI projects since 2017 and ran an AI startup from 2020 - 2022. Their expertise spans artificial intelligence, service design, and digital transformation, with a particular focus on the nonprofit sector. LinkedIn
Suzanne Begley is a strategic leader in nonprofit digital transformation, with over two decades of experience helping organisations amplify their social impact. As co-founder and former director of the award-winning agency Public Life, she has shaped digital strategies and services for prominent charities including Mencap, Macmillan Cancer Support and YoungMinds. LinkedIn